Search for Articles
Article Engineering in General
Evaluation of Revitalization Measures for Central City Areas Considering Changes in Human Flows
Journal Of Digital Life.2024, 4,4;
Received:September 9, 2024 Revised:October 3, 2024 Accepted:October 8, 2024 Published:November 21, 2024
- Atsushi Uechi
- Graduate School of Engineering and Science, University of the Ryukyus
- Daisuke Kamiya
- Faculty of Engineering, University of the Ryukyus
- Ryo Yamanaka
- Chuo Kensetsu Consultant, Co., Ltd.
- Daisuke Fukuda
- Graduate School of Engineering, The University of Tokyo
- Yoshiki Suga
- Regional Futures Research Co., Ltd.
Correspondence: d-kamiya@tec.u-ryukyu.ac.jp
This article has a correction. Please see:
Abstract
In recent years, a declining population and an aging and decline have led to a reduction in the vibrancy and economic activity of the city. Various regional cities have taken measures to revitalize their central city areas. However, they have yet to develop a method to quantify the effects of these measures. In this study, using a device equipped with Internet of Things (IoT), we obtained basic data on the number of visitors before the COVID-19 to quantify pedestrian traffic along Kokusai Street. Our findings revealed that the implementation of revitalization strategies led to a notable increase in the number of individuals visiting the area, as well as in the average duration of their stay. This suggests that the implemented measures have the potential to contribute to the revitalization of the area, thereby fostering the creation of a vibrant town center.
1. Introduction
Social changes in Japanese regional cities, including the advent of a super-aging society, population outflow, and urban sprawl resulting from unregulated development, have given rise to a pressing need to revitalize central city areas. In response to the COVID-19, a state of emergency was declared in numerous regions of the country, accompanied by requests for restrictions. The proliferation of novel lifestyles, such as the nationwide promotion of remote work and staggered work hours, has prompted a shift in commuting behavior (Hiroi, 2020).
To revitalize the central city area, it is necessary to increase visitor circulation through the utilization of public spaces, ensuring an appropriate layout of facilities, and establishing walking spaces. To increase visitor flow in the central city area, it is necessary to consider the perspectives of both users and operators of revitalization projects.
It is in the user’s interest to be able to visit facilities of their choice without impediments. Similarly, business operators are interested in encouraging visitors to explore the area more widely and visit a variety of facilities, rather than lingering in a particular location. In other words, an increase in the number of visitors, the duration of their stay in the central city area, and the number of locations they visit will stimulate economic activity in the area and facilitate the discovery of new attractions, with benefits extending to the entire central city area.
Accordingly, the construction of an evaluation index for the efficacy of urban center revitalization measures (hereinafter referred to as “revitalization measures”) and initiatives enables the tracking of changes over time and the comparison of numerical data of multiple municipalities, thereby facilitating appropriate evaluations by any interested party. Nevertheless, the implementation of quantitative and continuous surveys is challenging from both the technical and financial perspectives. Questionnaire-based surveys were conducted to assess the efficacy of the measures.
The advancements in sophisticated information and communication technology have precipitated the advent of transportation big data, which enables continuous observation of human behavior. Specifically, traffic big data, including cell phone base station data provided by cell phone operators, GPS data provided by application providers, and traffic system IC card data, are widely utilized. This study focuses on inexpensive and long-term observable Internet of Things (IoT) device data such as those collected by Wi-Fi packet sensors. In this study, we focus on IoT device data that are inexpensive and observable over an extended period.
A substantial body of research exists on survey methods employing Wi-Fi sensors, largely because of the simplicity of the equipment installation and the capacity to differentiate between building levels (Morimoto et al., 2015; Nishio et al., 2015). The standardization of the randomization of Media Access Control (MAC) addresses, which serve as unique identifiers for mobile terminals, has been established for the period after 2020 (Apple, 2020). Although there are some concerns about observing the same address for multiple days, numerous studies have demonstrated that it can effectively measure human flow in a small area, making it a valuable tool for understanding population flow in urban planning.
The “Smart Planning Practical Guide (2018),” published by the Ministry of Land, Infrastructure, Transport and Tourism, proposes a method for understanding human behavior derived from traffic big data and utilizing it to assess and evaluate the efficacy of revitalization strategies and urban development plans. By constructing an index that expresses the degree of human activity and using it to evaluate revitalization strategies, we can contribute to the sustainable development of the region of cities.
In this study, we constructed an index that expresses the degree of revitalization in a central city area using a sensor-based observation method and applied it to the central city area of Naha City, Okinawa Prefecture. The objective of this study was to capture changes in the behavior of visitors to the central city area before and after the COVID-19 and to elucidate the effects of revitalization measures.
The remainder of this paper is organized as follows: Section 2 summarizes previous studies and presents the positioning of this study. Section 3 presents an overview of the traffic flow survey method. In Section 4, we established the evaluation indicators for the revitalization of the central city area. In Section 5, we present the results obtained on applying the set evaluation indicators to the central city area of Naha City. The final chapter concludes the paper with a summary and suggestions for further study.
2. Positioning of Study in Relation to Previous Studies
2.1 Human Flow Analysis Using Wi-Fi Sensor-Based Measurement Methods
The extant literature on sensor-based human flow analysis is extensive, with a significant corpus of research papers published in Japan and elsewhere.
For example, Kusakabe et al estimated the waiting time of express bus users in the waiting room of a large-scale express bus terminal, “Basta Shinjuku,” based on the length of the observation period recorded by Wi-Fi sensors. A questionnaire survey was also conducted during the study period, the results of which indicated that the estimated waiting time derived from the Wi-Fi sensor was shorter than the actual waiting time as reported by respondents (Kusakabe er al., 2018). In another study, Wi-Fi sensors were used to estimate the boarding and alighting locations of paratransit users in Krabi, Thailand (Fukuda et al., 2017). Moreover, the use of sensors allows for an improved understanding of human movement patterns. This has been demonstrated in studies on tourist circulation behavior (Uechi et al., 2023) and the circulation behavior of people in a central city area (Imai et al., 2022).
Among the studies examining the assessment of revitalization strategies, many have employed questionnaires or statistical data on the advancement of strategies and the extent to which planned projects have been accomplished. To the best of our knowledge, the study conducted by Imai et al. was the only exception.
2.2 Human Flow Monitoring within the Central City Area of Naha City
Several monitoring studies have been conducted in the central city area of Naha City, Okinawa Prefecture, with the objective of assessing human flow. For example, pedestrian traffic volume was estimated by combining the results of an analysis of pedestrian attributes using camera images with data on human flow obtained from smartphones. (MLIT, 2021) Furthermore, the pedestrian traffic volume is measured once a year at multiple locations in the central city area. (Naha City, 2023)
Although pedestrian traffic volume surveys can provide accurate data on traffic volumes, they are only feasible in a limited section of the central city area and cannot capture changes in human behavior across the entire central city area. Furthermore, conducting surveys on a continuous basis is challenging because of logistical constraints such as securing survey personnel. Although it is feasible to obtain high-frequency and high-density data on human movements in an entire target area by leveraging data from smartphones, the data is often constrained to a limited temporal window owing to financial limitations.
Accordingly, we posit that this survey method, which is cost-effective and can be employed for prolonged observation, can inform the assessment of human movement patterns, thereby facilitating sustainable revitalization of the central city area.
2.3 Positioning of This Study
To comprehend the behavior of individuals in the central city and to quantify and assess the impact of revitalization initiatives, it is imperative to conduct sustained surveys. We posit that human flow monitoring in the urban areas through this approach will not only address the concerns previously identified by Naha City, but also provide valuable insights for human flow monitoring in the central city, which plays a pivotal role in the PDCA cycle.
In this study, the flow of people to the central city of Naha was monitored. By comparing the data observed before and after the onset of COVID-19, we were able to elucidate changes in people’s behavior in the central city area and construct an index that expresses the degree of human activity in the central city area. This allowed us to clarify the effects of the revitalization measures quantitatively.
3. Survey Overview and Data Cleansing Methodology
3.1 Overview of Naha Central City Area
The central area of Naha City, the subject of this study, had been a vibrant tourism and commerce hub in Okinawa Prefecture since the Second World War, with Kokusai Street serving as this primary commercial artery (Naha City, 2018). However, following the COVID-19 pandemic, the number of tourists declined significantly, and stores along Kokusai Street were forced to close or cease operations due to the declaration of a state of emergency and other factors. This led to a gradual exodus of residents from the prefecture. The “Kokusai Street Transit Mall” (hereinafter referred to as “TM”) and the “Kokusai Street Marche” (hereinafter referred to as “Marche”) have been held regularly on Sundays as revitalization measures, and the number of tourists and residents is gradually recovering. However, there is no system for measuring these effects.
3.2 Equipment Installation Point and Duration Setting
The survey was conducted from August 1 to September 20, 2017, prior to the advent of COVID-19, and from July 22, 2020, to April 4, 2022, after COVID-19, by installing the sensors depicted in Fig.1 at Naha Airport and in the central district of Naha City, as illustrated in Fig.2. The survey equipment collects probe requests (hereinafter referred to as “PRs”) periodically sent from electronic devices such as smartphones. It also accumulates data on MAC addresses, which are unique identification numbers assigned to each terminal, the PR time, and the electric field strength. MAC addresses are anonymized and recorded concurrently with the acquisition of PR time, and data cannot be collected for electronic devices whose Wi-Fi function is deactivated. In this study, anonymized MAC addresses are designated as unique IDs (hereinafter referred to as “IDs”) and are analyzed using PR reception times and location information.
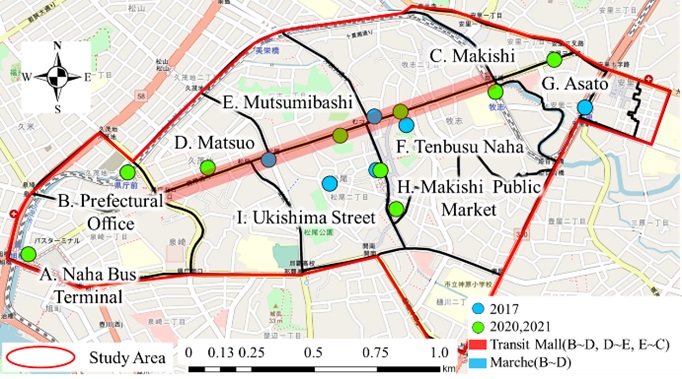
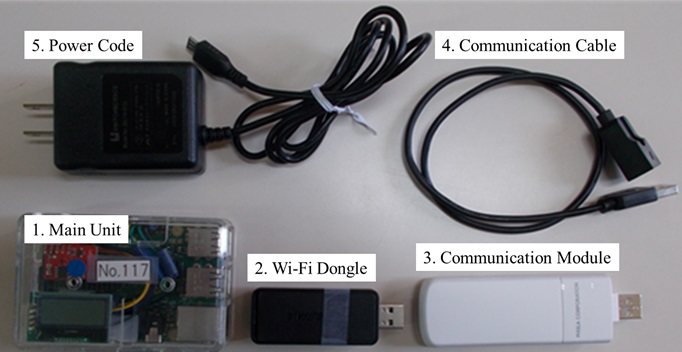
In this study, to monitor human flow before and after the COVID-19 pandemic, the three periods shown in Table 2 were set based on the status of the issuance of requests for COVID-19 pandemic restrictions during the study period shown in Table 1 and the implementation of the “TM” and “Marche” as revitalization measures (Naha City, 2020-2021). To measure the effect of revitalization measures based on human flow data and data observed only on Sundays during study the period were used.
3.3. Data Cleaning
In accordance with the methodology proposed by Tanaka et al., we assigned a threshold to the starting point of observation and the difference in the number of observation days to provide information on the attributes of in-prefecture and out-of-prefecture residents. This is because both tourists and residents of the prefecture visit the central area of Naha City (Tanaka, 2019).
IDs that were first or last observed at a location other than Naha Airport, and those with a difference of seven days or more in the number of observation days during the entire period, were defined as “in-prefecture data”, and henceforth referred to as “prefectural residents”. Table 3 presents the numbers of prefectural residents identified. Despite the impact of MAC address randomization on data from 2020 onwards, the daily number of IDs remained consistent with that observed in 2017 prior to the emergence of COVID-19. The number of IDs was also observed in 2020 because of the TM and the Marche. Consequently, we posit that the data can be used to comprehend behavioral patterns within a central city area. We contend that these data can be used to gain insight into the behavior of individuals within the central city area. In this study, we focus our analysis on the behavior of prefectural residents, who have been moving away from shopping areas in recent years.
Table 1. Data Acquisition Period
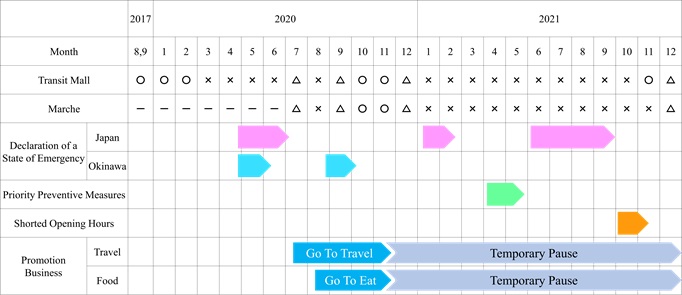
Table 2. Term Setting

Table 3. Number of IDs by Period

4. Construction of Evaluation of Revitalization Measures Indicators for the Central City Area
In this Section, we present an index that quantitatively expresses the degree of human activity in the central city area of Naha City, as described in Section 1. We then compared the data obtained from the sensors before and after the onset of the COVID-19 pandemic to determine the effects of the revitalization measures. The concept and calculation method of the index are as follows:
4.1 Establishment of Evaluation Indicators and Their Approach
a) Visitation Rate
The behavior of individuals in the central city area is thought to have undergone notable alterations contingent on the introduction of promotional initiatives and events at commercial establishments. Consequently, we established the ratio of the number of IDs observed in the vicinity of the sensor installation point as an evaluation metric and conducted a quantitative assessment by comparing the outcomes before and after the onset of COVID-19.
b) Number of Observation Points
The implementation of revitalization measures will enhance the mobility of individuals within the central city area. The enhancement of mobility facilitated the promotion of people’s movement, which significantly contributed to the vibrancy of the town. Consequently, we established the number of observations made by the sensors for each ID as an evaluation index and conducted a quantitative evaluation.
c) Length of Stay Time
The implementation of revitalization measures and events is significantly related to the increase in the time people spend not only in the places they visit, but also in the area. Because an increase in the time spent in the central city area contributes greatly fostering a lively town center, we considered the time spent in the central city area as a whole and the time spent in the vicinity of the sensor installation point as evaluation indices and conducted a quantitative evaluation.
d) Transit Time
TM and Marche have been implemented in the central city area of Naha City as revitalization measures to create attractive street spaces. The implementation of these measures is anticipated to foster the creation of a vibrant town center and contribute to the local economy by attracting people to stores and event sites along the roadside. Consequently, transit time was established as an evaluation index, and a quantitative evaluation of the measures was conducted.
4.2 Calculation Method of Evaluation Indicators
a) Visitation Rate
The visitation rate by location was defined as the ratio of the number of IDs observed by location to the number of IDs observed by period. This ratio was calculated and evaluated for each location at which the sensors were installed. This study focused on the behavior of prefectural residents visiting the central city area of Naha City. Therefore, the visitation rate was calculated for nine points, excluding Naha Airport.
b) Number of Observation Points
The number of sensors observed for each ID was recorded over the course of the day. Subsequently, the ratio of the number of IDs observed in each period was calculated, allowing the evaluation of changes in the migration of IDs to the central area of Naha City.
c) Length of Stay Time
In this paper, we present a methodology for calculating the length of stay in an entire central city area and the length for a single sensor.
First, the time elapsed between the last and first observations of the day by the sensors installed throughout the central city area was calculated, representing the length of stay in the central city area during the daytime. However, in this case, there is a possibility that IDs that are continuously located in the central city area as well as IDs that only make brief stops in the central city area may be calculated as having spent the same amount of time in the central city area. Accordingly, to prevent an overestimation of the time spent in the central city area, a threshold was established for the interval between PR acquisition times by the sensor, spanning a range of 30-180 min. This value is approximately equivalent to the time it takes to walk through the study area, and the change in behavior was observed by varying the threshold in a sensitivity analysis manner.
For example, when the designated time was set to 30 min, if no PR was observed by the sensors throughout the central city area for a period exceeding 30 min, the visitor was deemed to have departed from the central city area. Consequently, the surplus time was deducted from the length of stay within the central city area.
This methodology enables the assessment of the length of stay time within the central city area.
Subsequently, the interval between the final and initial observations on a given day at a sensor was calculated, representing the length of stay at each designated day/location. The time spent by each sensor per day and location is calculated based on the data acquisition range of the sensor (the maximum range is approximately 300 to 400 m, but this study assumes a radius of approximately 100 m) and the walking speed of a person (this study assumes a speed of 80m/min). The time spent using the same sensor was determined to be a minimum of 2 min and 30s, and those determined to be passing were eliminated. By setting a threshold value for the interval between the PR acquisition times, it is possible to evaluate areas that are becoming increasingly crowded.
d) Transit Time
In the central area of Naha City, the implementation of TM has thus far been limited to the red section (B-C) along Kokusai Street, as illustrated in Fig.1. Concurrently, a Marche was held in the blue section (B-D) following the COVID-19 outbreak.
The transit time in this section was calculated by first extracting the OD pairs that commenced and concluded at the sensors in the TM section and then calculating the transit time between the sensors. Subsequently, the linear distance between the sensors was measured, and the time taken to walk at a speed generally defined as fast walking (in this paper, a speed of 93m/min is assumed (Meiji Yasuda Health Development Foundation, 2017)) was calculated (hereinafter referred to as “fast walking time”). If the time interval between the sensors is less than the fast-walking time, it is assumed that the individual in question has used a mode of transportation other than walking and the data of this individual are thus removed from the data set. Finally, the average transit time the IDs spent in the section with revitalization measures implemented was calculated. This allows for the measurement of the effect of revitalization measures on walking time.
5. Results of Application of Evaluation Indicators
5.1 Visitation Rate
Fig.3 illustrates the outcomes of implementing the evaluation indicators described in Section 4.1 a). Consequently, the visitation rate declined in most locations compared with the pre COVID-19 period. However, the visitation rates for “F. Tenbusu Naha,” “H. Makishi Public Market,” and “I. Ukishima Street” in the central city area exhibited the highest rates in 2020 following the COVID-19 period. It is postulated that the visitation rate for “H. Makishi Public Market” increased because of the relocation to a provisional storefront, which was completed in 2020. Conversely, the visitation rate for the entire central city area exhibited a declining trend, indicating that COVID-19 may have diminished the number of visits by the prefecture’s residents.
The visitation rate was calculated as the ratio of the number of IDs that visited the locations where the sensors were installed. Furthermore, by limiting the departure and arrival points, it is possible to estimate the general means of transportation and quantitatively grasp changes in the range of visitor behavior.
5.2 Number of Observation Points
Fig.4 illustrates the outcomes of utilizing the evaluation indicator conceptualized in Section 4.1 b). The ratio of the number of IDs observed at two or more locations within the central city area demonstrated that the average number of observation points increased following the 2020 COVID-19 outbreak, which occurred one year later. The elevated average value in 2020 compared to the pre COVID-19 period indicates that the implementation of revitalization measures had a discernible impact on enhancing circulation.
However, the observed increase in the average value in 2021 can be attributed to the waning awareness of the need to refrain from certain activities, which may have resulted from repeated requests for restrictions.
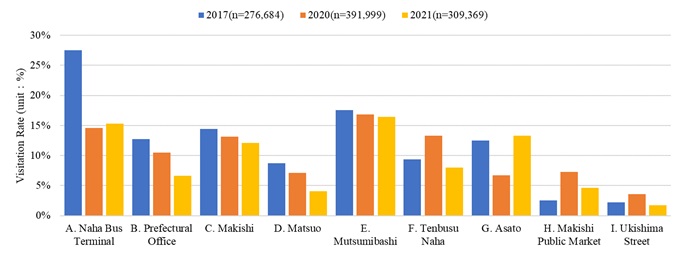
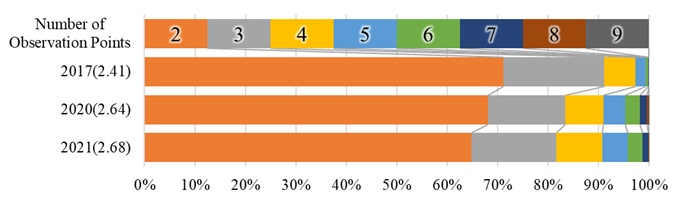
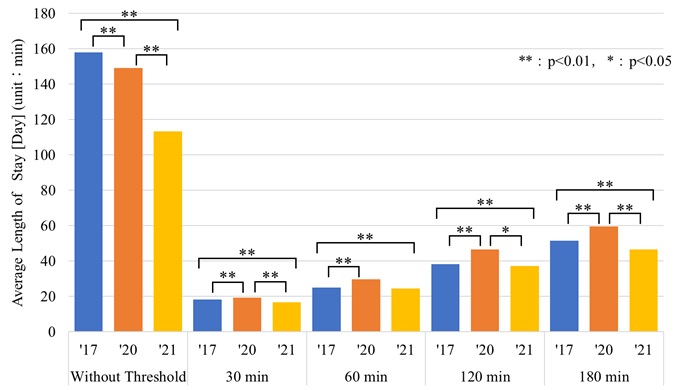
5.3. Length of Stay
a) Length of Stay [Day]
The mean daily length of stay was calculated using the indicator described in Section 4.1 c). The results are presented in Fig.5. A comparison of the results indicates that, except for the case where no threshold was set for the interval between PR acquisition times, the year 2020 had the longest mean daily length of stay, and the year 2021 had a mean daily length of stay similar to or shorter than that of the year 2017, the year before the COVID-19. This suggests that the implementation of revitalization measures may have a certain effect on increasing the length of stay time walking in the city.
b) Length of Stay Time [Day・Point]
Fig.6 illustrates the outcomes of implementing the indicator developed in Section 4.1 c). In this study, the threshold for the interval between the PR acquisition times was set to 30 min, which represents the strictest condition. The results demonstrate that the mean duration of visits to the locations designated as “D. Matsuo”, “E. MutsumiBashi”, and “F. Tenbusu Naha”, which are situated at the center of Kokusai Street, exhibited an increase during the period of the COVID-19, whereas the mean duration of visits to other locations exhibited a decrease. This suggests that although the average length of stay increased across the entire central city area, it increased only in the central area by location. This indicates that the Kokusai Street area may have experienced a decline in vibrancy due to the impact of COVID-19. The results demonstrate the potential of evaluating the characteristics of each area using this indicator.
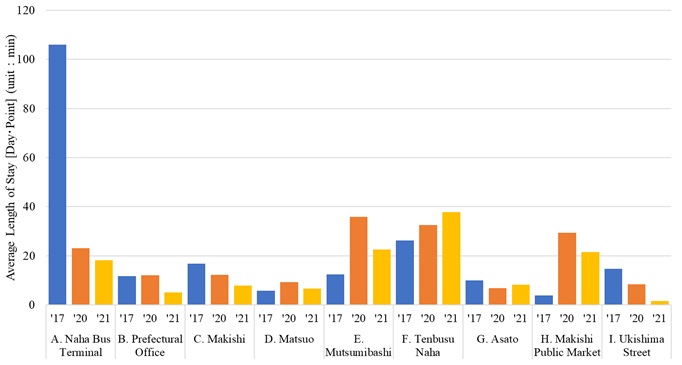
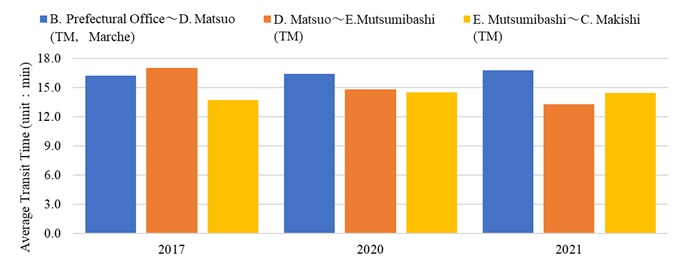
5.4 Transit Time
Fig.7 illustrates the outcomes of utilizing the evaluation indicator conceptualized in Section 4.1 d). A comparison of the results pertaining to TM implementation, categorized into three sections, revealed that in 2020, when the activation measures were initiated, the transit time was the longest in the section overlapping with the Marche implementation section.
The implementation of the Marche, a revitalization measure, was observed to have a certain degree of effect on the increase in dwell time. This indicates that it is possible to grasp the trend of people’s behavior in the central city area by calculating and comparing the transit time between sections.
6. Conclusion
The objective of this study is to elucidate the alterations in human traffic patterns before and after the COVID-19 in the central city area of Naha City. Furthermore, it seeks to quantify the impact of central city area revitalization measures based on monitoring of human traffic. In developing this study, an evaluation index was incorporated, building upon previous studies (Uechi, 2022). The findings of this study, along with potential future avenues for research, are presented below.
In this study, we developed an evaluation index for the revitalization of the central city area by monitoring human flow using IoT devices and applied it to the central city area of Naha City. Furthermore, we quantitatively analyzed the actual conditions of movement within the central city area before and after the COVID-19, as well as the effects of the revitalization measures.
Consequently, despite a decline in the number of visits by residents of the prefecture to the downtown area, the number of visitors and the duration of their stay in the town increased. These findings indicate that the implementation of revitalization measures may have a certain degree of effect on the creation of a lively town center, which had been lost due to the COVID-19, by stimulating people’s behavior, which had been reduced due to the COVID-19.
Furthermore, it is believed that this survey method can be applied to the quantitative and continuous monitoring of the actual movement status and evaluation of revitalization measures in a narrow spatial scale, such as in a central city area. Although Wi-Fi data was used in this study, it is anticipated that the randomization of MAC addresses will become increasingly challenging in the future. Consequently, it will be feasible to utilize Bluetooth Classic data, in which the MAC address remains constant, to conduct a similar observation.
In addition to the analysis results, it is imperative to maintain a continuous monitoring of the effects of revitalization measures, such as TM and Marche, on the revitalization of central city areas in the context of the ongoing Coronavirus pandemic, as well as in the post-pandemic era. Although this survey was completed in April 2022, we will continue to refine the evaluation indices by integrating them with diverse forms of transportation-related big data, including app location data and Wi-Fi access point data. Furthermore, we will conduct analyses that consider spatial hierarchies, such as wide and narrow areas, and examine the correlation between these indices and satisfaction levels and store sales through questionnaires. Furthermore, an effort will be made to enhance the sophistication of the evaluation indices by examining the relationship with satisfaction levels and store sales through questionnaire surveys.
Author Contributions
Conceptualization, D.K. and A.U.; methodology, D.K.; writing—original draft preparation, D.K. and A.U.; writing—review and editing, D.K. and A.U.; project administration, D.K.; funding acquisition, D.K.
All authors have read and agreed to the published version of the manuscript.
Funding
This research was supported by the Japan Society for the Promotion of Science (JSPS) Grants-in-Aid for Scientific Research (JP22H00222, JP23H00197, and JP24K15538).
Informed Consent Statement
The sensor utilized in this measurement employs Wi-Fi radio waves to anonymize and encrypt terminal information (MAC address) embedded within Wi-Fi signals emitted by smartphones and other devices, subsequently transforming it into secure identification data for enumeration purposes. The data obtained is solely utilized for the purpose of identifying MAC addresses; it does not involve the interception or recording of any personal information, including names, addresses, or the content of communications conducted via Wi-Fi. The acquired data undergoes comprehensive analysis to ensure the anonymity of individuals and is employed to ascertain regional travel trends through the statistical examination of aggregate values over a specified time frame.
Acknowledgments
The data utilized in this study were procured through the Research and Development of Technology for Improving the Quality of Road Policy (Research and Development of “Data-driven” Traffic Demand and Spatial Management Using ETC2.0 Probe Information, etc., PI: Daisuke Fukuda) and the Regional Road Economics Strategy Research Group, Okinawa.
The data were obtained by the Research Group on Regional Road Economy Strategies, Okinawa. The authors extend their sincerest gratitude to the following organizations.
Conflicts of Interest
The authors declare no conflict of interest.
References:
Apple, (2021,02,18). Wi-Fi Privacy, https://support.apple.com/ja-jp/guide/security/secb9cb3140c/web (in Japanese, accessed 2022.04.05)
Fukuda, D. et al. (2017). Estimation of Paratransit Passengers Boarding/Alighting Locations Using Wi-Fi based Monitoring: Results of Field Testing in Karabi City, Thailand, Journal of the Eastern Asia Society for Transportation Studies, 12, pp.2151-2169, https://doi.org/10.11175/easts.12.2151
Hiroi, U. (2020). A study on the Effect of Japanese-style lockdown on Self-restraint Request for COVID-19. Journal of the City Planning Institute of Japan, 55(3), pp.902-909. (in Japanese) https://doi.org/10.11361/journalcpij.55.902
Imai, R. et al. (2022). TRAFFIC FLOW SURVEY IN CENTRAL SHIZUOKA CITY USING IoT DEVICES, proceedings of Infrastructure Planning, 66. (in Japanese)
Kusakabe, K. et al. (2018). Estimation of bus passengers’ waiting time at a coach terminal with Wi-Fi MAC addresses, International Steering Committee for Transport Survey Conference, 32, pp.62-68, https://doi.org/10.1016/j.trpro.2018.10.012
Meiji Yasuda Health Development Foundation, (2017). How fast is fast walking?, https://www.my-kenshin.jp/viewpdf.php?id=20170704153827&tmp=455680e00ec5e58c4a4ae210975a9baf (accessed 2023.01.01)
Ministry of Land, Infrastructure, Transport and Tourism, (2018). Smart Planning Guide-New Town planning Based on Individual Data-. (in Japanese, accessed 2022.02.25)
Ministry of Land, Infrastructure, Transport and Tourism, (2021). PLATEAU, Human flow analysis using a combination of different monitoring techniques, https://www.mlit.go.jp/plateau/use-case/uc20-006/ (accessed 2023.09.20)
Morimoto, T. et al. (2015). Method of pedestrian and traffic flow analysis using Wi-Fi packet sensor. Forum on Information Technology 2015, 14(4), pp.505-511. (in Japanese) https://www.ieice.org/publications/conference-FIT-DVDs/FIT2015/data/pdf/O-034.pdf
Naha City, (2018). Naha City Kokusai Street Transit Mall Vision (Future Vision) Report, https://www.city.naha.okinawa.jp/admin/cityhall/sosiki/sosiki/bukyoku/keizaikankoubu/nahamati.files/houkokusyo_nahasikokusaidooritorannjitto.pdf (accessed 2021.12.10)
Naha City, (2023). 2023 Central city area of Naha city Shopping Street Traffic Survey, https://www.city.naha.okinawa.jp/admin/cityhall/sosiki/sosiki/bukyoku/keizaikankoubu/nahamati.files/R5tstukouryoutyousahoukoku.pdf (accessed 2023.09.20)
Naha City Kokusai Street Shopping Street Promotion Association Federation, (2020-2021). Past transit miles, https://naha-kokusaidori.okinawa/en/eventinfo/transitmall.html (accessed 2022.09.28)
Nishio, N. et al. (2015). Research and Development on Wi-Fi Packet-based Anonymous human Flow Analysis System at “Umekita”, ICT Innovation Forum 2015, Strategic Information and Communications R&D Promotion Programme (SCOPE), https://www.soumu.go.jp/main_content/000393923.pdf (in Japanese)
Tanaka, K. et al. (2019). Analysis of the Tourists’ Travel Behavior Using Wi-Fi Packet Sensor: A Case Study in Okinawa Main Island, Journal of Japan Society for Fuzzy Theory and Intelligent Informatics, 31(6), pp.876-886, https://doi.org/10.3156/jsoft.31.6_876 (in Japanese)
Uechi, A. et al. (2022). ANALYSIS OF VISITATION CHARACTERISTICS IN THE CENTRAL AREA OF NAHA CITY - CONSIDERING COVID-19 -, proceedings of Infrastructure Planning, 66. (in Japanese) Uechi, A. et al. (2023). Analysis of the Relationship between the Travel Behavior of inbound Tourists and Web Information: A Case Study in Yaeyama Islands, Journal of Informatics and Infrastructure, 4(3), pp.646-655, (in Japanese) https://doi.org/10.11532/jsceiii.4.3_646
Relevant Articles
-
An attempt to realize digital transformation in local governments by utilizing the IT skills of information science students
by Edmund Soji Otabe - 2025,4
VIEW -
Fundamental Study on Detection of Dangerous Objects on the Road Surface Leading to Motorcycle Accidents Using a 360-Degree Camera
by Haruka Inoue - 2025,S1
VIEW -
Wildlife Approach Detection Using a Custom-Built Multimodal IoT Camera System with Environmental Sound Analysis
by Katsunori Oyama - 2025,S2
VIEW -
A Study on the Development of a Traffic Volume Counting Method by Vehicle Type and Direction Using Deep Learning
by Ryuichi Imai - 2025,S4
VIEW