Search for Articles
Article Electrical and Electronic Engineering Interdisciplinary Sciences
Heart Rate Variability Indices May Change Accompanying Cognitive Skills Improvement in eSports Tasks
Journal Of Digital Life.2022, 2,4;
Received:November 8, 2021 Revised:December 27, 2021 Accepted:February 28, 2022 Published:March 8, 2022
- Kazuki Hisatsune
- Department of Computer Science and Electrical Engineering, Graduate School of Science and Technology, Kumamoto University
- Toshihide Otsuki
- Department of Computer Science and Electrical Engineering, Graduate School of Science and Technology, Kumamoto University
- Goichi Hagiwara
- Department of Human Science, Kyushu Sangyo University
- Hirohisa Isogai
- Department of Human Science, Kyushu Sangyo University
- Toshitaka Yamakawa
- Division of Informatics and Energy, Faculty of Advanced Science and Technology, Kumamoto University
Correspondence: yamakawa@cs.kumamoto-u.ac.jp
Abstract
Electronic sports (eSports) is becoming an increasingly popular subject of research with progress in the video game industry. However, the relationship between eSports and cognitive skills and heart rate variability (HRV) indices is not fully understood. Therefore, in this study, we analyzed changes in HRV indices in 20 healthy adult men while playing eSports and evaluated improvement in their cognitive skills before and after playing eSports using the Stroop test. The subjects were divided into two groups: 10 subjects who were trained in eSports for at least 1 hour a day, 5 days a week for 6 weeks, and 10 subjects who were not trained. The results indicated that subjects in the training group tended to have improved cognitive skills. In addition, in the group that temporarily improved their cognitive skills by playing eSports, similar changes were observed in HRV indices during eSports play, suggesting a parasympathetic nervous system dominance. Thus, it is suggested that the observed HRV changes were accompanied by the temporary improvement in cognitive skills induced by eSports tasks.
1. Introduction
The term “electronic sports (eSports)” refers to organized, competitive forms of video games that can be played individually or in a team and can be viewed by spectators either in-person or via streaming services (Brevers et al.,2020; Hamari & Sjöblom., 2017). With a history of more than 20 years, eSports has rapidly developed into a profitable industry because of its large number of competitors and the development of professional gaming leagues (Chikish et al., 2019; Kim et al., 2020; Wagner, 2006). With the progress in the video game industry, the popularity of eSports as a research subject has increased (Reitman et al., 2020). eSports is used as a countermeasure against post-traumatic stress disorder, schizophrenia, neurodegenerative diseases that reduce the volume of the hippocampus and frontal area, (Kühn et al., 2014) and as a digital therapy for attention deficit hyperactive disorder (Kollins et al., 2020). However, the drawbacks of eSports include reduced sleep due to the use of electronic devices (Hysing et al., 2015), depression, and decreased academic achievement due to video game addiction (Brunborg et al., 2014). Toth et al. (2019) highlighted that for further development of eSports, adequate research with a focus on cognitive skills is essential. Hagiwara et al. (2020) analyzed the level of concentration using an electroencephalogram (EEG) before and during a racing-type eSports task in 20 male university students (mean age ± standard deviation: 21.4±1.7) who trained for at least 1 hour a day for 4 days a week for 6 months. In addition, their cognitive skills were measured using the Stroop test before and after playing eSports. The results showed that eSports improved both concentration and cognitive skills. In addition, Anguera et al. (2013) studied the relationship between video game playing and cognitive skills in elderly people. The results showed that elderly people who were trained to play video game for 1 hour a day, 3 days a week, for 4 weeks, had an increased power ratio in the theta wave band of the EEG, which reflects increased subconscious attention and concentration while playing video game, compared with elderly people who were not trained. Furthermore, improved performance on subsequent cognitive skills tests was reported. Many other previous studies have reported that eSports enhance a variety of cognitive functions (Bediou et al., 2018; Oei & Patterson., 2013; Qiu et al., 2018) focusing on the brain activity recorded using EEG as a bioindicator. However, few studies have focused on heart rate variability (HRV) analysis and cognitive skills during eSports activities.
HRV is an accurate and noninvasive method for measuring autonomic nervous functions of the cardiovascular system (Malik et al., 1996) and provides objective assessment of psychological states, such as detection of a driver falling asleep while driving (Fujiwara et al., 2018), stress (Castaldo et al., 2015; Kim et al., 2018), and emotional classification (Chen et al., 2019). In addition, previous studies have reported that cognitive skills are related to stress and HRV indices (Fuentes-García et al., 2019; Hansen et al., 2004; Hilgarter et al., 2021; Rodrigues et al., 2018). Furthermore, unlike EEG measurements, HRV indices can be measured using a wearable device, which improves usability. Therefore, measuring the changes in HRV indices while playing eSports may capture the changes in cognitive skills.
Therefore, this study tested the hypothesis that HRV indices could change accompanying the improvement in cognitive skills induced by eSports.
2. Materials and Methods
2.1 Assessment of cognitive skills using Stroop test
The Stroop test is a neuropsychological test used to assess cognitive skills. The test consists of a congruent task in which the participants are asked to respond as accurately and quickly as possible to four colors, namely red, blue, green, and yellow, and an incongruent task in which the participants are asked to respond as accurately and quickly as possible to the ink color of a word whose color and meaning are incongruent (Stroop, 1935; Van der Elst et al., 2006). The increase in reaction time when responding to a task in which the letter color and meaning are mismatched compared to the congruent task is called the Stroop test effect and is used as an index to measure the inhibitory function of controlling behavior, which is a cognitive skill (Lemay et al., 2004; MacLeod., 1991; Stroop, 1935). Inhibitory function is the ability to suppress the dominant action or thought in a given situation (Martínez-Loredo et al., 2017). Previous studies have reported that the reaction time obtained from the Stroop test is a reliable and stable measurement of inhibitory function (Martínez-Loredo et al., 2017). In this study, we used two Stroop tests to measure the cognitive skills: a written version (Shin Stroop Kensa II, Tokyo Physical, Fukuoka, Japan) (ST) and an app version (Hacaro–Stroop test, Digital Standard, Osaka, Japan) (STapp) based on previous studies (Hagiwara et al., 2020). An iPad Pro (12.9 inches, Apple, California, USA) was used to conduct the STapp. The congruent task in STapp is a task in which a colored circle (red, blue, green, or yellow) is displayed 20 times and the user is expected to identify the color of the circle as accurately and quickly as possible. In the incongruent task, a character with a mismatched color and meaning (e.g. “Red” printed with blue ink) is displayed 20 times and the user is expected to identify the color of the ink as accurately and quickly as possible. In both the tasks, the number of correct answers to the 20 questions and time elapsed are displayed as the results. The written ST is a task with four items, each of which must be answered as accurately and quickly as possible within a time limit of 60 seconds. Test items 3 and 4, which correspond to the congruent and incongruent tasks of STapp, were used in the ST. In this study, the number of correct answers for each item was obtained from the ST, and the total reaction time for each of the 20 questions in each item was obtained in the STapp and used as a measure of cognitive skill.
2.2 RRI measurement and HRV analysis using a wearable system
The method used to calculate HRV indices from the R-R interval (RRI) (the time between two adjacent R waves of the QRS complex in the electrocardiogram (ECG)) is an accurate and noninvasive method for measuring the autonomic nervous functions (Malik et al., 1996). In this study, we evaluated autonomic nervous activity during eSports play, Stroop test, by continuously measuring RRIs using an original RRI telemeter shown in Fig. 1(a) and calculating the HRV indices using the dedicated smartphone application shown in Fig. 1(b) (Yamakawa et al., 2020). The size of the RRI telemeter is 75 × 35 mm (excluding the three ECG leads) with a thickness of 12 mm (42.3 g). It has a battery life of 45 hours of continuous use. In addition, the device is equipped with an automatic gain optimization function (to perform appropriate amplification according to the subject’s ECG waveform) and a gain readjustment function (to respond to a decrease in the R wave detection rate). These features enable longitudinal and stable RRI measurement without expert knowledge in physiological measurements (Yamakawa et al., 2013). The measured RRI data are transmitted from the telemeter to a smartphone using Bluetooth LE and are analyzed for HRV using the application shown in Fig. 1 (b). In this study, we calculated eight HRV indices: four time-domain indices: mean of RRI (mean NN), standard deviation of the RRI (SDNN), root mean square of the differences of adjacent RRIs (RMSSD), and the number of pairs of adjacent RRIs whose difference is greater than 50 ms within a given length of time (NN50) and four frequency-domain indices: variance of RRI (Total Power, TP), power of the low-frequency band of 0.04–0.15 Hz (LF), power of the high-frequency band of 0.15–0.4 Hz (HF), and the LF to HF ratio (LF/HF) (Malik et al., 1996). Because the HRV indices are obtained by statistical or frequency analysis of a 3-minute moving window, any outlier contamination in the RRI adversely affects the subsequent 3-minute indices. Therefore, in this study, we have used the same RRI real time correction technique as in previous studies (Yamakawa et al., 2020) to replace the outlier RRIs. Additionally, the application consists of a function to record the user’s physical status. During the measurement, the buttons “Eating,” “In Bed,” “Got up,” “Restroom,” “etc.,” and “Stop” are displayed along with the average heart rate, as seen in Fig. 1 (b). In this experiment, during the measurement (e.g., resting, STapp) was recorded by tapping the “etc.” button during the measurement.
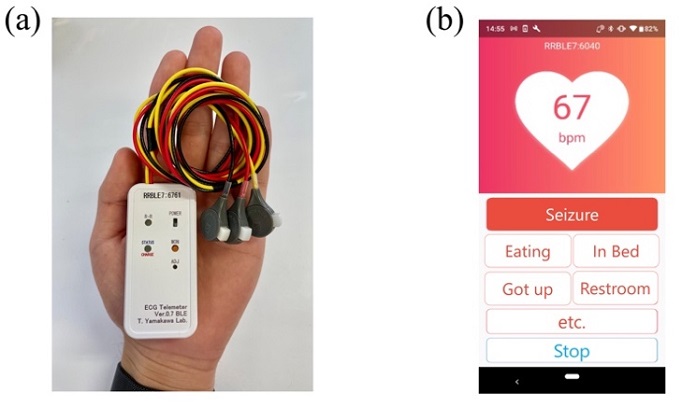
2.3 Experimental procedure
This experiment was conducted on 20 healthy adult male subjects (mean age ± standard deviation: 21.9 ± 0.7). Informed consent was obtained from all the subjects involved in the study. The experiment was conducted using the procedure shown in Fig. 2, referring to previous studies (Hagiwara et al., 2020). Subjects were randomly divided into two groups of 10 each: a training group that received at least 1 hour of racing eSports training per day for at least 5 days a week for 6 weeks and a non-training group that did not receive any eSports training. Before starting the training, the subjects in the training group had to solve ST example tasks for 10 s each followed by ST. Next, they were asked to play racing-type eSports (MARIO KART 8 DELUXE, Nintendo, Kyoto, Japan) using a dedicated game console (Nintendo Switch, Nintendo, Kyoto, Japan) for the aforementioned period. After the Six-week training period, the training and non-training groups were asked to solve ST example tasks for 10 s each and then ST was performed. The RRI telemeter was then attached to the subjects using a standard lead II and the connection between the RRI telemeter and a dedicated smartphone application was checked to ensure that the data were transmitted and received correctly. Next, the subjects were made to sit on a chair in the resting position with eyes open for 8 min (Rest). Then, the first STapp (STapp1) was conducted. The subjects played the single-time attack mode (TA) of Mario Kart 8 Deluxe three times. TA is a mode in which a single player, who can use only three acceleration devices, runs three laps around a course and competes for time. Immediately following the end of the TA was completed, the second STapp (STapp2) was conducted. After the completion of the STapp2, the subjects were instructed to sit on a chair in the resting position with eyes open for 10 min (Recovery), which was the recovery time. Next, the subjects played the computer versus mode (COM) three times. In COM, a total of 12 characters run three laps around a course while using various items (e.g., acceleration devices, jamming devices) and compete for the first position. Immediately following the end of the COM, the third STapp (STapp3) was conducted, and the experiment was completed. The course used was Mario Kart Stadium, class was 150 cc, and characters and machines were chosen by the subjects.
The study was conducted in accordance with the guidelines of the Declaration of Helsinki and approved by the Ethics Review Committee of the School of Advanced Science and Technology, Kumamoto University (protocol code R2-2).

3. Data analysis and statistical test
3.1 Stroop test
The ST was used to compare subject’s cognitive skills with and without training. The combined results of the first ST of the training and non-training groups were used as the pre-training data. The second ST of the training group was used as the post-training data. Based on previous studies (Ikeda, 2013a, b), all data were confirmed to be normally and equally distributed using the Shapiro–Wilk and Bartlett tests. The unpaired t-test was conducted using the pre-training and post-training data to determine whether the cognitive skills were improved by training. The significance level was set at 0.05.
The STapp was used to compare acute changes in cognitive skills before and after the eSports task. The combined results of the training and non-training groups were used as the data for each STapp. Since the data were confirmed to be normally and equally distributed, a one-way analysis of variance (ANOVA) followed by Tukey’s test was conducted on the three groups of STapp1, STapp2, and STapp3 individually to determine whether there were acute changes in cognitive skills before and after the eSports task. The significance level was set at 0.05.
3.2 HRV indices
To examine the trend of the HRV indices obtained from the RRI, HRV indices were plotted as time-series data. The mean values of HRV indices in each condition were calculated for the top 10 (Top10) and bottom 10 (Bottom10) subjects when the subjects were arranged in order of the difference between the total reaction times of STapp1 and STapp3. The Shapiro–Wilk test showed that these data could not be assumed to be normally distributed. Therefore, a comparison of the mean values of the Rest and COM states of the Top 10 was conducted using Wilcoxon’s signed-rank test. Bottom10 also compared Rest and COM as well as Top10. In addition, the difference between the Rest and COM values of the HRV indices, and the difference between the total reaction times of STapp1 and STapp3 for the data of all subjects, were used to calculate the Spearman’s correlation coefficient and an uncorrelation test was conducted. The significance level was set at 0.05.
4. Results
4.1 Stroop test
Table 1 shows the results for the ST and STapp. The results of the unpaired t-test for the ST showed that there was no significant difference in the number of correct answers in both congruent and incongruent tasks between pre- and post-training ST (congruent task: t(28)=−0.20, p>0.05; incongruent task: t(28)=−1.22, p>0.05). Although the difference was not significant, the number of correct answers to the ST incongruent task was higher in post-training than in pre-training.
The results of the ANOVA for the total reaction time of STapp showed that there was no significant difference between all STapp in the congruent task (F(2, 57)=1.70, p>0.05). However, the results of the Tukey’s test for the total reaction time of STapp showed that there was a significant difference in the performance of incongruent tasks in STapp1 and STapp3 (p<0.05). The ANOVA results of the number of correct answers of the STapp showed that there was no significant difference among all three groups of the STapp (congruent task: F(2, 57)=0.23, p>0.05; incongruent task: F(2, 57)=0.84, p>0.05). The STapp results showed that cognitive skills were significantly improved temporarily after playing COM. Seven subjects of the Top10 group were from the training group.
4.2 HRV indices
The mean values and standard deviations of SDNN, RMSSD, and LF/HF of Rest and COM for the Top10 and Bottom10 are shown in Table 2. SDNN and RMSSD have been reported as parasympathetic HRV measures related to cognitive skills, while LF/HF has only been reported to be an indicator of sympathetic system (Castaldo et al., 2015; Hilgarter et al., 2021; Roos et al., 2017; Schumann et al., 2019; Thayer et al., 2012).
For the Top10, the values of SDNN and RMSSD during COM were higher than those at Rest; the results of the Wilcoxon’s signed-rank test showed that the difference was statistically significant (p<0.05). In contrast, for Bottom10, the values of SDNN and RMSSD during COM were lower than those at Rest; although the results of the Wilcoxon’s signed-rank test showed that the difference was not statically significant (p>0.05). However, correlation analysis of the difference between the Rest and COM values of these HRV indices and the difference in total reaction time between STapp1 and STapp3 showed no significant correlation between the two (SDNN: r=−0.26, RMSSD: r=−0.37, LF/HF: r=−0.12, p>0.05).
Fig. 3 shows the time-series plots of RMSSD and LF/HF for the subjects with the most and least difference between the total reaction times of STapp1 and STapp3. The RMSSD of the subject with the greatest difference exhibited an increasing trend and LF/HF exhibited a decreasing trend during COM, and vice-versa for the subject with the least difference. Additionally, these trends were observed in each of the Top10 (similar to the former) and Bottom10 (similar to the latter) groups.
Table 1. Results of congruent and incongruent tasks for ST and STapp. The values in the table represent mean ± standard deviation. *p < 0.05 between the total reaction time of STapp1 and STapp3 incongruent task.
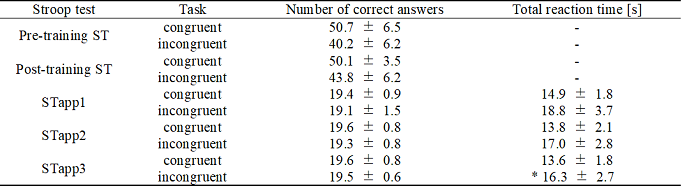
Table 2. HRV indices at Rest and COM for Top10 and Bottom10. The values in the table represent mean ± standard deviation. *p < 0.05 between Rest and COM HRV indices value.

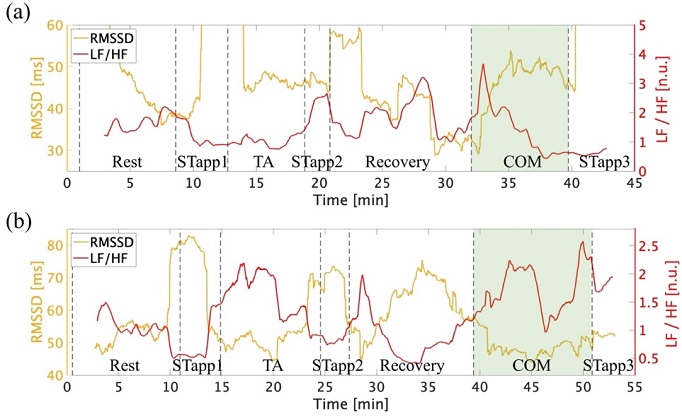
The green band indicates the play of COM.
5. Discussion
5.1 Stroop tests
5.1.1 ST
The results of the ST indicated that only training did not improve the number of correct answers on ST. However, a trend of improvement was observed in the incongruent task, although the unpaired t-test showed it was not statistically significant. Hagiwara et al. (2020) reported that the results of the congruent and incongruent tasks of the Stroop test were significantly improved by training. In their study, the training period for the training group was 6 months, while the training period in the present study was 6 weeks. This difference in the training period may have caused the difference in Stroop test results.
5.1.2 STapp
The results of ANOVA showed that the number of correct answers in any STapp was over 19 questions, which was not significantly different. These results suggest that the subjects did not make lazy choices for each STapp, and responded with appropriate deliberation. In addition, the results of Tukey’s test showed a significant difference in the total reaction time between STapp1 and STapp3 in the incongruent task. These results suggest that the reason for the significant decrease in total reaction time in STapp3 compared with that in STapp1 is due to the decrease in thinking time caused by the temporary improvement in cognitive skills by playing COM. However, STapp3 was the third STapp in the experimental procedure. Therefore, we cannot deny the possibility that the total reaction time was significantly shortened because of the subjects’ familiarity with the STapp. The obtained result supports those found in a previous study (Anguera et al., 2013; Bediou., 2018; Hagiwara et al., 2020), which showed that cognitive skills are improved by playing eSports. However, the results did not show that playing TA significantly reduced the total reaction time of the STapp which is different from the results of a previous study (Hagiwara et al., 2020). COM requires the subject to process more information than TA mode because during COM, the subject has to process interference from other players. The total reaction time was much shorter compared to STapp1 may be related to the difference in the amount of information processed during play in COM and TA modes.
5.2 HRV indices
RMSSD reflects the beat-to-beat variability and has been reported to be the primary time-domain feature used to estimate vagal changes reflected in HRV (Shaffer et al., 2014). It has also been reported that a relationship exists between the vagus nerve and frontal lobe activity, and subjects with poor results in cognitive tasks involving the frontal lobe have lower values in several HRV features (Forte et al., 2019; Muthukrishnan et al., 2017; Thayer et al., 2012). In the study by Hagiwara et al. (2020), the power of the EEG measured from the frontal lobe was significantly greater while playing eSports. In addition, Anguera et al. (2013) showed an increase in EEG activity measured from the frontal lobe during the playing of video games. Table 2 shows that the Top10’s RMSSD of the COM increased significantly compared with that of the rest. The higher RMSSD values in the Top10 than in the resting state may reflect activation of the frontal lobe.
Recently, it has been suggested that playing video games may enhance inhibitory function (Bediou., 2018; Hutchinson et al., 2016; Liu et al., 2019). People with high inhibitory function have been reported to have higher HRV indices in the parasympathetic nervous system (Roos et al., 2017; Schumann et al., 2019). In addition, a previous study reported that the parasympathetic nervous system was activated during the Stroop test in a group with good test results (Iwasa, 2012). From Table 2, Top10’s SDNN and RMSSD of the COM were significantly increased compared to the resting state, which may suggest the improvement in inhibitory function induced by playing eSports.
Fig. 3 (a) shows that the RMSSD values exhibit an increasing trend during COM. In addition, LF/HF values exhibit a decreasing trend. These trends were observed at the Top10. It is suggested that these results reflect activation of the parasympathetic nervous system caused by a temporary increase in frontal lobe activation and inhibitory function.
Previous studies have reported the potential of playing video games to both induce and reduce stress (Aliyari et al., 2018, Aliyari et al., 2020). Stress is inhibited when concentration and attention to playing video games are increased. When playing video games suppresses stress, subsequent cognitive skills are improved (Aliyari et al., 2020). Similarly, cognitive skills are hampered under stressful conditions (Castaldo et al., 2015; Rodrigues et al., 2018). Under stress, SDNN and RMSSD values are significantly decreased and LF/HF values, which represent sympathetic nerve activity, are significantly increased (Castaldo et al., 2015). Table 2 shows that the Bottom10’s RMSSD and SDNN of the COM decreased compared with that of rest. Furthermore, Fig. 3 (b) shows that the RMSSD value exhibits a decreasing trend during COM. In addition, the LF/HF value exhibits an increasing trend. These trends were observed in the Bottom10. These results suggest that Bottom10 may have been stressed by playing eSports. As a result, cognitive skills are likely to be temporarily reduced or unchanged. In this way, a time-series plotting of RMSSD and LF/HF may be able to assess the temporary improvement of cognitive skills induced by eSports play.
In this study, seven of the Top10 subjects belonged to the training group. In the study by Hagiwara et al. (2020), 6 months of training for people who belonged to an eSports club resulted in a significant temporary improvement in cognitive skills. On the other hand, in this study, the participants were not members of the eSports club and had a short training period. This suggests that habitual practice and proficiency in eSports may contribute to the temporary improvement in cognitive skills induced by eSports play.
One of the limitations of this study is that, in this experiment, the age of the subjects was 21.9 ± 0.7 years, which is a very narrow range; hence, we cannot determine if the above discussion is valid for different age groups. In this experiment, the subjects were divided into two groups, namely training, and non-training groups, and the number of subjects in each group was 10, which could be an insufficient sample size. Increasing the sample size and optimally normalizing the inter-participant variance of the HRV indies will improve the correlation analysis and evaluation of quantitative effects. In addition, we did not conduct a questionnaire survey to ascertain the regularity with which the subjects usually played eSports; hence, we cannot deny the possibility that there were subjects in the non-training group who played eSports to the same extent as the ones in the training group.
6. Conclusions
This study verified the hypothesis that there are HRV indices that change accompanying the improvements in cognitive skills induced by eSports. The Top10’s SDNN and RMSSD values for the parasympathetic nervous system during eSports play were significantly higher than those recorded at rest. Furthermore, time-series plots of RMSSD and LF/HF data during eSports play suggested an activation of the parasympathetic nervous system. On the other hand, the Bottom10’s SDNN and RMSSD values for the parasympathetic nervous system during eSports play were decreased compared to the at-rest condition. Furthermore, time-series plots of RMSSD and LF/HF data during eSports play suggested sympathetic nervous system activation. These results suggest that the temporary improvement in cognitive skills induced by eSports may be accompanied by the HRV changes in the parasympathetic indices. The time-series plotting examples of RMSSD and LF/HF suggested the possibility to develop a prediction system for cognitive skill improvement during eSports based on real-time analysis of HRV indices.
Author Contributions
Conceptualization: K.H., G.H. and T.Y.; methodology: K.H., T.O., G.H., H.I. and T.Y.; software: K.H., T.O., G.H., H.I. and T.Y.; validation: K.H., T.O. and T.Y.; formal analysis: K.H., T.O. and T.Y.; investigation: K.H., T.O. and T.Y.; resources: K.H., T.O., G.H., H.I. and T.Y.; data curation: K.H., T.O. and T.Y.; writing—original draft preparation: K.H.; writing—review and editing: K.H. and T.Y.; visualization: K.H., T.O. and T.Y.; supervision: T.Y.; project administration: K.H. and T.Y.; All authors have read and agreed to the published version of the manuscript.
Funding
This research received no external funding.
Institutional Review Board Statement
The study was conducted according to the guidelines of the Declaration of Helsinki, and approved by the Ethics Committee of the School of Advanced Science and Technology, Kumamoto University (protocol code R2-2 and date of approval December 3, 2020).
Informed Consent Statement
Informed consent was obtained from all subjects involved in the study.
Conflicts of Interest
The authors declare no conflict of interest.
References
Aliyari, H., Sahraei, H., Daliri, M. R., Minaei-Bidgoli, B., Kazemi, M., Agaei, H., Sahraei, M., Hosseini, S., Hadipour, M. M., Mohammadi, M., & Dehghanimohammadabadi, Z. (2018). The beneficial or harmful effects of computer game stress on cognitive functions of players. Basic and Clinical Neuroscience, 9(3), 177–186. https://doi.org/10.29252/nirp.bcn.9.3.177
Aliyari, H., Sahraei, H., Erfani, M., Mohammadi, M., Kazemi, M., Daliri, M. R., Minaei-Bidgoli, B., Agaei, H., Sahraei, M., Seyed Hosseini, S., Tekieh, E., Salehi, M., & Farajdokht, F. (2020). Changes in cognitive functions following violent and football video games in young male volunteers by studying brain waves. Basic and Clinical Neuroscience, 11(3), 279–288. https://doi.org/10.32598/bcn.9.10.335
Anguera, J. A., Boccanfuso, J., Rintoul, J. L., Al-Hashimi, O., Faraji, F., Janowich, J., Kong, E., Larraburo, Y., Rolle, C., Johnston, E., & Gazzaley, A. (2013). Video game training enhances cognitive control in older adults. Nature, 501(7465), 97–101. https://doi.org/10.1038/nature12486
Bediou, B., Adams, D. M., Mayer, R. E., Tipton, E., Green, C. S., & Bavelier, D. (2018). Meta-analysis of action video game impact on perceptual, attentional, and cognitive skills. Psychological Bulletin, 144(1), 77–110. https://doi.org/10.1037/bul0000130
Brevers, D., King, D. L., & Billieux, J. (2020). Delineating adaptive esports involvement from maladaptive gaming: a self-regulation perspective. Current opinion in psychology, 36, 141–146. https://doi.org/10.1016/j.copsyc.2020.07.025
Brunborg, G. S., Mentzoni, R. A., & Frøyland, L. R. (2014). Is video gaming, or video game addiction, associated with depression, academic achievement, heavy episodic drinking, or conduct problems? Journal of Behavioral Addictions, 3(1), 27–32. https://doi.org/10.1556/JBA.3.2014.002
Castaldo, R., Melillo, P., Bracale, U., Caserta, M., Triassi, M., & Pecchia, L. (2015). Acute mental stress assessment via short term HRV analysis in healthy adults: A systematic review with meta-analysis. Biomedical Signal Processing and Control, 18, 370–377. https://doi.org/10.1016/j.bspc.2015.02.012
Chen, Y. C., Hsiao, C. C., Zheng, W. D., Lee, R. G., & Lin, R. (2019). Artificial neural networks-based classification of emotions using wristband heart rate monitor data. Medicine, 98(33), e16863. https://doi.org/10.1097/MD.0000000000016863
Chikish, I., Carreras, M., & García, J. (2019). eSports: a new era for the sports industry and a new impulse to research in sports (and) economics, in García, J. (Ed.), Sports (and) Economics. FUNCAS Social and Economic Studies, 7, 477–508.
Forte, G., Favieri, F., & Casagrande, M. (2019). Heart rate variability and cognitive function: a systematic review. Frontiers in Neuroscience, 13, 710. https://doi.org/10.3389/fnins.2019.00710
Fuentes-García, J. P., Villafaina, S., Collado-Mateo, D., de la Vega, R., Olivares, P. R., & Clemente-Suárez, V. J. (2019). Differences between high vs. low performance chess players in heart rate variability during chess problems. Frontiers in Psychology, 10, 409. https://doi.org/10.3389/fpsyg.2019.00409
Fujiwara, K., Abe, E., Kamata, K., Nakayama, C., Suzuki, Y., Yamakawa, T., Hiraoka, T., Kano, M., Sumi, Y., Masuda, F., Matsuo, M., & Kadotani, H. (2019). Heart Rate Variability-Based Driver Drowsiness Detection and Its Validation With EEG. IEEE transactions on bio-medical engineering, 66(6), 1769–1778. https://doi.org/10.1109/TBME.2018.2879346
Hagiwara, G., Kawahara, I., & Kihara, S. (2020). [An attempt to verify the positive effects of esports: Focusing on concentration and cognitive skill.] Journal of Japan Society of Sports Industry, 30(3), 239–246. https://doi.org/10.5997/sposun.30.3_239
Hamari, J., & Sjöblom, M. (2017). What is eSports and why do people watch it?. Internet research.
Hansen, A. L., Johnsen, B. H., Sollers, J. J., Stenvik, K., & Thayer, J. F. (2004). Heart rate variability and its relation to prefrontal cognitive function: the effects of training and detraining. European Journal of Applied Physiology, 93(3), 263–272. https://doi.org/10.1007/s00421-004-1208-0
Hilgarter, K., Schmid-Zalaudek, K., Csanády-Leitner, R., Mörtl, M., Rössler, A., & Lackner, H. K. (2021). Phasic heart rate variability and the association with cognitive performance: A cross-sectional study in a healthy population setting. PLOS ONE, 16(3), e0246968. https://doi.org/10.1371/journal.pone.0246968
Hutchinson, C.V., Barrett, D.J., Nitka, A., Raynes, K.: Action video game training reducesthe simon effect. Psychon. Bull. Rev. 23, 587–592 (2016). https://doi.org/10.3758/s13423-015-0912-6
Hysing, M., Pallesen, S., Stormark, K. M., Jakobsen, R., Lundervold, A. J., & Sivertsen, B. (2015). Sleep and use of electronic devices in adolescence: results from a large population-based study. BMJ Open, 5(1), e006748. https://doi.org/10.1136/bmjopen-2014-006748
Ikeda, I, (2013a). [For those who use statistical tests without understanding them II] (in Japanese). Kagaku To Seibutsu, 51(6), 408–413. https://doi.org/10.1271/kagakutoseibutsu.57.562
Ikeda, I, (2013b). [For those who use statistical tests without understanding them III] (in Japanese). Kagaku To Seibutsu, 51(7), 483–495. https://doi.org/10.1271/kagakutoseibutsu.51.483
Iwasa Y, (2012). [The effect of frontal lobe function test on heart rate variability in adolescents] (in Japanese). The Journal of Nursing Investigation, 10(1, 2), 28–35.
Kim, H. G., Cheon, E. J., Bai, D. S., Lee, Y. H., & Koo, B. H. (2018). Stress and heart rate variability: a meta-analysis and review of the literature. Psychiatry Investigation, 15(3), 235–245. https://doi.org/10.30773/pi.2017.08.17
Kim, Y., Nauright, J., & Suveatwatanakul, C. (2020). The rise of E-Sports and potential for Post-COVID continued growth. Sport in Society, 23(11), 1861–1871. https://doi.org/10.1080/17430437.2020.1819695
Kühn, S., Gleich, T., Lorenz, R. C., Lindenberger, U., & Gallinat, J. (2014). Playing Super Mario induces structural brain plasticity: gray matter changes resulting from training with a commercial video game. Molecular Psychiatry, 19(2), 265–271. https://doi.org/10.1038/mp.2013.120
Kollins, S. H., DeLoss, D. J., Cañadas, E., Lutz, J., Findling, R. L., Keefe, R., Epstein, J. N., Cutler, A. J., & Faraone, S. V. (2020). A novel digital intervention for actively reducing severity of paediatric ADHD (STARS-ADHD): a randomised controlled trial. The Lancet Digital Health, 2(4), e168–e178. https://doi.org/10.1016/S2589-7500(20)30017-0
Lemay, S., Bédard, M. A., Rouleau, I., & Tremblay, P. L. (2004). Practice effect and test-retest reliability of attentional and executive tests in middle-aged to elderly subjects. The Clinical Neuropsychologist, 18(2), 284–302. https://doi.org/10.1080/13854040490501718
Liu, X., Liao, M., & Dou, D. (2019). Video Game Playing Enhances Young Children’s Inhibitory Control. HCI.
MacLeod C. M. (1991). Half a century of research on the Stroop effect: an integrative review. Psychological Bulletin, 109(2), 163–203. https://doi.org/10.1037/0033-2909.109.2.163
Malik, M., Bigger, J. T., Camm, A. J., Kleiger, R., Malliani, A., Moss, A., & Schwartz P. (1996). Heart rate variability. Standards of measurement, physiological interpretation, and clinical use. European Heart Journal, 17(3), 354-381. https://doi.org/10.1093/oxfordjournals.eurheartj.a014868
Martínez-Loredo, V., Fernández-Hermida, J. R., Carballo, J. L., & Fernández-Artamendi, S. (2017). Long-termreliability and stability of behavioral measures among adolescents: The Delay Discounting and Strooptasks. Journal of Adolescence, 58, 33–39. https://doi.org/10.1016/j.adolescence.2017.05.003
Muthukrishnan, S. P., Gurja, J. P., & Sharma, R. (2017). Does heart rate variability predict human cognitive performance at higher memory loads? Indian Journal of Physiology and Pharmacology, 61(1), 14–22.
Oei, A. C., & Patterson, M. D. (2013). Enhancing cognition with video games: a multiple game training study. PLOS ONE, 8(3), e58546. https://doi.org/10.1371/journal.pone.0058546
Qiu, N., Ma, W., Fan, X., Zhang, Y., Li, Y., Yan, Y., Zhou, Z., Li, F., Gong, D., & Yao, D. (2018). Rapid Improvement in Visual Selective Attention Related to Action Video Gaming Experience. Frontiers in Human Neuroscience, 12, 47. https://doi.org/10.3389/fnhum.2018.00047
Reitman, J. G., Anderson-Coto, M. J., Wu, M., Lee, J. S., & Steinkuehler, C. (2020). Esports research: a literature review. Games and Culture, 15(1), 32–50. https://doi.org/10.1177/1555412019840892
Rodrigues, S., Paiva, J. S., Dias, D., Aleixo, M., Filipe, R. M., & Cunha, J. (2018). Cognitive impact and psychophysiological effects of stress using a biomonitoring platform. International Journal of Environmental Research and Public Health, 15(6), 1080. https://doi.org/10.3390/ijerph15061080
Roos, L. E., Knight, E. L., Beauchamp, K. G., Berkman, E. T., Faraday, K., Hyslop, K., & Fisher, P. A. (2017). Acute stress impairs inhibitory control based on individual differences in parasympathetic nervous system activity. Biological psychology, 125, 58–63.
Schumann, A., Köhler, S., Brotte, L., & Bär, K. J. (2019). Effect of an eight-week smartphone-guided HRV-biofeedback intervention on autonomic function and impulsivity in healthy controls. Physiological measurement, 40(6), 064001. https://doi.org/10.1088/1361-6579/ab2065
Shaffer, F., McCraty, R., & Zerr, C. L. (2014). A healthy heart is not a metronome: an integrative review of the heart’sanatomy and heart rate variability. Frontiers in Psychology, 5, 1040. https://doi.org/10.3389/fpsyg.2014.01040
Stroop, J. R. (1935). Studies of interference in serial verbal reactions. Journal of Experimental Psychology, 18(6),643–662. https://doi.org/10.1037/h0054651
Toth, A. J., Kowal, M., & Campbell, M. J. (2019). The color-word stroop task does not differentiate cognitive inhibition ability among esports gamers of varying expertise. Frontiers in Psychology, 10, 2852. https://doi.org/10.3389/fpsyg.2019.02852
Thayer, J. F., Ahs, F., Fredrikson, M., Sollers, J. J., & Wager, T. D. (2012). A meta-analysis of heart rate variability and neuroimaging studies: implications for heart rate variability as a marker of stress and health. Neuroscience & Biobehavioral Reviews, 36(2), 747–756. https://doi.org/10.1016/j.neubiorev.2011.11.009
Van der Elst, W., Van Boxtel, M. P., Van Breukelen, G. J., & Jolles, J. (2006). The Stroop color-word test: influence of age, sex, and education; and normative data for a large sample across the adult age range. Assessment, 13(1), 62–79. https://doi.org/10.1177/1073191105283427
Wagner, M. (2006). On the scientific relevance of esport, in Proceedings of the 2006 International Conference on Internet Computing and Conference on Computer Game Development, CSREA Press, Las Vegas, Nevada, 437–440.
Yamakawa, T., Fujiwara, K., Kano, M., Miyahima, M., Suzuki, Y., Maehara, T., Ohta, K., Sasano, T., Matsuura, M., & Matsushima, E. (2013). Development of a wearable HRV telemetry system to be operated by non-experts in daily life, 2013 Asia-Pacific Signal and Information Processing Association Annual Summit and Conference, 1–4. https://doi.org/10.1109/APSIPA.2013.6694225.
Yamakawa, T., Miyajima, M., Fujiwara, K., Kano, M., Suzuki, Y., Watanabe, Y., Watanabe, S., Hoshida, T., Inaji, M., & Maehara, T. (2020). Wearable epileptic seizure prediction system with machine-learning-based anomaly detection of heart rate variability. Sensors, 20(14), 3987. https://doi.org/10.3390/s20143987
Relevant Articles
-
An attempt to realize digital transformation in local governments by utilizing the IT skills of information science students
by Edmund Soji Otabe - 2025,4
VIEW -
Fundamental Study on Detection of Dangerous Objects on the Road Surface Leading to Motorcycle Accidents Using a 360-Degree Camera
by Haruka Inoue - 2025,S1
VIEW -
Wildlife Approach Detection Using a Custom-Built Multimodal IoT Camera System with Environmental Sound Analysis
by Katsunori Oyama - 2025,S2
VIEW -
A Study on the Development of a Traffic Volume Counting Method by Vehicle Type and Direction Using Deep Learning
by Ryuichi Imai - 2025,S4
VIEW