Search for Articles
How much do you bid? Answers from ChatGPT in first-price and second-price auctions
Journal Of Digital Life.2023, 3,11;
Received:August 14, 2023 Revised:August 23, 2023 Accepted:September 5, 2023 Published:October 4, 2023
- Toshihiro Tsuchihashi
- Faculty of Economics, Daito Bunka University
Correspondence: tsuchihasi@ic.daito.ac.jp
Abstract
This research examines the feasibility of using ChatGPT as a subject in an auction experiment. The author will also test the idea of giving ChatGPT a “persona” to participate as a human. Without personas, the author finds overbidding in the FPA and slight underbidding in the SPA; the FPA results are consistent with prior studies, but the SPA results differ. Given the persona as an excellent economics student, ChatGPT bid close to the theoretical prediction in the FPA but significantly underbid in the SPA. These results raise questions about using LLM-based AI subjects in auctions.
keywords:
1. Introduction
Experimental research has been significant in economics since Vernon L. Smith established experimental laboratory methods for empirical economic analysis. Experiments have been widely used, especially in auction theory. It would be exciting if artificial intelligence (AI) experiments could tell us the same about human behavior and decision-making as experiments with human subjects. Using AI as a subject[1] has the potential to alleviate the handling of subjects and financial problems faced by researchers. Motivated by this possibility, this study explores the feasibility of a chatbot experiment using ChatGPT in an auction experiment.
Auction theory assumes that a bidder maximizes his/her payoff. The payoff is the monetary valuation, or private value, of the auctioned item minus the payment. Bidders bid privately and simultaneously, and the bidder with the highest bid wins.
The payment rules for first-price auctions (FPA) and second-price auctions (SPA) differ: in FPA, the winning bidder pays the highest bid; in SPA, the winning bidder pays the second highest bid (the loser’s highest bid). This study examines whether ChatGPT bids in the FPA and SPA are consistent with human bids. In addition, this research tries a novel idea of giving ChatGPT a “persona” and using it as a human subject.
The corpus used by ChatGPT, which is based on the large language model (LLM), would include terms related to decision-making in auctions.[2] Using the relationship between those terms, ChatGPT could answer the specific amounts of bids and how bids are determined in auctions.
Using ChatGPT as an experimental subject involves three critical caveats. First, it is known that ChatGPT has a bias.[3] Second, the corpus used by ChatGPT for learning should contain many false beliefs, messages, and errors, and GPT may not distinguish them from true beliefs and messages.[4] Third, while experimental economics provides monetary rewards to subjects, it is unclear whether the “rewards” given to ChatGPT motivate GPT to search for the correct answer (perhaps it does not).
This research revealed the following results: Without persona, ChatGPT’s responses in first-price auctions (FPA) aligned with human subject bids, and in second-price auctions (SPA), they matched theoretical predictions. However, with a persona as an economics student, the responses in FPA were similar to theoretical predictions, and in SPA, they were closer to bids from subjects with more experience in auctions. Thus, the author must withhold judgment on employing ChatGPT as a subject.
These results differ from Aher et al. (2023) and Holton (2023), who reported that they replicated the results of experimental studies of ultimatum and dictator games.
2. Experimental Design and Procedures
Bidders participate in a series of auctions (called sessions). Each session consists of 20 auctions (called rounds). Each bidder is informed of the auction outcomes for each round, after which the experiment proceeds to the next round. For each round, five bidders (Bidder 1, Bidder 2, Bidder 3, Bidder 4, and Bidder 5) will place bids. Of these, ChatGPT is assigned the role of Bidder 1. This study used ChatGPT-3.5 (free version), released by OpenAI in November 2022.
At the beginning of the round, bidders observe private values. Following Kagel & Levin (1993), this research assumes that private values are uniformly distributed over [$0.00, $28.30]. Throughout the experiment, this research uses identical realizations randomly generated a priori shown in Table 1.[5]
Table 1. Private values
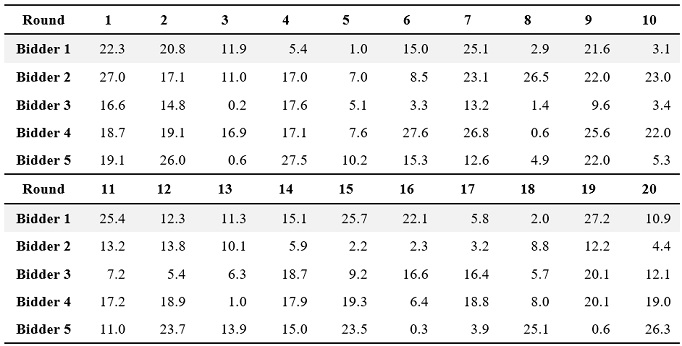
Except for ChatGPT, with a valuation of , the other four bidders bid according to theoretically optimal strategies as follows.[6]

Given the five bids, the bidder with the highest bid wins the auction and obtains a payoff of private value minus the amount paid. The losers earn nothing.
The author creates a “prompt” and conducts an interactive experiment using ChatGPT as the subject. The author informs ChatGPT of each round’s private value and asks for its bid. Then, the author notifies ChatGPT of the bid outcome, the reward earned, and the bids of the other four bidders. This research experimented over two days, with four sessions in total. On the first day, the auction order was FPA and SPA, and on the second day, it was SPA and FPA.
3. Experimental Results
3.1. Auctions without Persona
Figure 1 shows the results of bids in FPA and SPA. The values are standardized to [0,1]. The equations in the figure are linear approximations of the bidding functions.
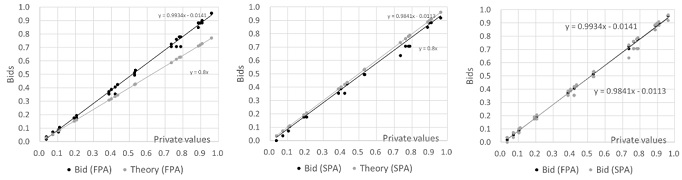
Finding 1: In both FPA and SPA, ChatGPT bids slightly below its private value, leading to an overbid in FPA and a slight underbid in SPA.[7]
Finding 2: ChatGPT’s bids remain consistent within each session, and auction results do not influence subsequent rounds’ answers.
In the previous experiments, both FPA and SPA showed a trend of overbidding (Cox et al., 1982; Cox et al., 1988; Güth et al., 2003; Kagel & Levin, 1993). In finding 1, the FPA results are consistent with previous studies.[8] Finding 2 suggests ChatGPT might not learn from auction outcomes.[9]
In the FPA, ChatGPT often provides a “reason for choosing that bid” response along with the bid.[10] An interesting example is: “One commonly used strategy in auctions is to bid an amount close to your own valuation.” However, this strategy is neither theoretically optimal nor demonstrated by human subjects in laboratory experiments.
In auction theory, an auction outcome is efficient if and only if the bidder with the highest valuation wins the auction. Table 2 shows the efficiency and the rewards obtained by ChatGPT in the FPA and the SPA. In Table 2, Efficiency is calculated as the ratio of (a) the results that the bidder with the highest valuation wins to (b) the total results: Efficiency=(a)/(b). Result shows (c) the average of payoffs ChatGPT earns in 20 rounds, and Theory shows (d) the average of payoffs ChatGPT would earn if it followed the theoretically optimal bidding strategy given by Formula (1). Result/Theory is calculated as the ratio of (c) to (d): Result/Theory=(c)/(d). The efficiency of FPA and SPA are 90% and 100%, respectively. The reason for the loss of efficiency in the FPA is that ChatGPT won in rounds that ChatGPT could not have won. Focusing on the rewards, however, it can be seen that in the FPA, ChatGPT earned only 7-13% of the theoretical prediction. This is because the degree of overbidding is so great that the rewards from winning an auction are tiny. The rewards earned by ChatGPT in the SPA are consistent with theoretical predictions. In addition, the auction results were utterly efficient. The high efficiency achieved is because ChatGPT’s responses are almost equal to the private values, automatically realizing a payoff in theory.
Table 2. Payoff and efficiency in FPA and SPA
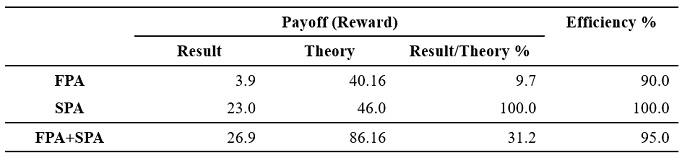
3.2. Auctions with Persona
In subsection 3.1, ChatGPT consistently underbids in SPA, while its bids in the FPA and SPA are almost identical. These results are inconsistent with the findings that human subjects tend to overbid in SPA and that bids in FPA are lower than bids in SPA (Cox et al., 19822; Cox et al., 1988; Güth et al., 2003; Kagel & Levin, 1993). ChatGPT’s answers differed from human subjects, possibly due to lacking guidelines on “human-like behavior.” To explore this, the author next gives ChatGPT a “persona” and asks for responses.
Persona is “a precise descriptive model of users, what he wishes to accomplish, and why” (Cooper & Reimann, 2003, p. 55). There have already been attempts to create personas using generative AI, and Butler (2023) proposed a specific method for creating personas using ChatGPT. Emily Thompson,[11] created by ChatGPT, is an economics student with a GPA of 3.8 to 4.0 and “approaches problem-solving with a logical and methodical mindset.” ChatGPT answered the bid as Emily. The experimental procedure is the same as in Section 2. Figure 2 shows the results of the bids in FPA and SPA.
Finding 3: When ChatGPT assumes the persona of Emily, bids in FPA align closely with theoretical predictions, while SPA bids tend to underbid. Average SPA bids are slightly higher than FPA bids, deviating from previous experimental results.
Finding 4: With Emily’s persona, ChatGPT’s bids show inconsistency throughout the session. In the FPA, bids approach the theoretical predictions in later rounds.
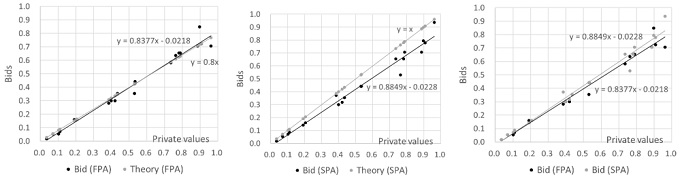
In Figure 3, the vertical axis represents the ratio of Emily’s bid to the theoretically predicted bid (above 1 indicates overbidding), and the horizontal axis represents the round number. The figure reveals bid inconsistency in both FPA and SPA, but late-round bids in FPA seem to approach the theoretical predictions (ratio near 1). This suggests that, with Emily’s persona, ChatGPT may have learned from previous rounds and integrated that knowledge into its bidding strategy, unlike when it was not given a persona.
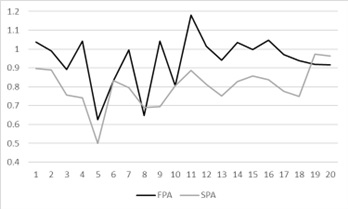
Table 3 shows the results of the efficiency and the rewards Emily earned in the FPA and the SPA. The bid in the FPA was almost optimal, and the auction outcomes were thoroughly efficient. Although the slight overbid lowered the payoff, ChatGPT earned as high as 88.7% of the theoretical prediction. On the other hand, an underbid in the SPA was inefficient, resulting in a slight loss in both rewards and efficiency.
Table 3. Payoff and efficiency in FPA and SPA
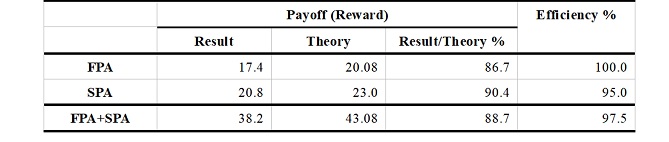
4. Concluding Discussion
Unlike the previous research, this research’s findings cast doubt on the usefulness of using LLM-based AI subjects. One possible explanation for these differences is that previous studies (Aher et al., 2023; Holton, 2023) involved ChatGPT making judgments about the tradeoff between fairness and efficiency, while in my auction experiments, the chatbot made bid decisions based on “logical thinking” in decision making. Interestingly, AI, often regarded as proficient in computation, yields similar results to human subjects in experiments challenging the concept of values but differs significantly in answering “optimal bids” compared to humans. Moreover, even if a model such as the LLM reproduces human behavior in an experiment that has already been studied, it is not sure that it will behave the same way as humans in future experiments that have yet to be conducted.
Whatever the reason, the difference between this study and Holton (2023) suggests that some experiments are better suited to employing LLM-based AIs as subjects in experiments than others. It is necessary to conduct chatbot experiments in many frameworks in the future to verify what kind of experiments are suitable for AI. Also, using a different persona than “Emily” would likely change the ChatGPT responses. Thus, it would be interesting to explore personas that achieve results similar to those of existing experiments.
The logic and thinking principle that ChatGPT uses to derive bids from given valuations is currently a black box, and it would be an exciting study to elucidate it. However, this issue is beyond the scope of this paper and awaits future research.
In the field of auction theory, many experimental studies observed that actual bidding differed from theoretical predictions. Many theories have subsequently emerged to explain these observations, for example, risk aversion (Cox et al., 1988) and regret (Engelbrecht-Wiggans & Katok, 2008) were proposed to explain overbidding. If multiple studies confirm this study’s findings in the future, it is expected that theoretical studies on the issue of “how ChatGPT’s bids are determined” may follow. In this sense, this study provides a first step in discussing the actual use of AI subjects.
Funding
This research received no external funding.
Institutional Review Board Statement
Not applicable.
Informed Consent Statement
Not applicable.
Data Availability Statement
All chat histories are available at the URLs below.
FPA-1: https://chat.openai.com/share/e57c1020-b185-4c23-ad55-ce5a299562da
FPA-2: https://chat.openai.com/share/48defac1-96e6-458e-9f81-38128c25d2d9
SPA-1: https://chat.openai.com/share/b80e15a0-c4f3-43a8-8be0-f3237f413251
SPA-2: https://chat.openai.com/share/d521117a-88e7-4442-840d-6723791a6a45
FPA (Emily): https://chat.openai.com/share/e17bb5bf-5a67-471b-950f-915f27d660e8
SPA (Emily): https://chat.openai.com/share/44db2708-f3d5-49ab-bda2-ffa8d5234bfc
Acknowledgments
The authors are truly indebted to the attendees at the Lunch Time Seminar at Daito Bunka University for their helpful comments and suggestions, and for their invaluable guidance. Any remaining errors are, of course, our own responsibility.
Appendix. Detailed Description of Emily Thompson.
Name: Emily Thompson
Personality Traits:
Analytical: Emily has a natural inclination towards analyzing complex economic concepts and enjoys critically evaluating data and trends. She possesses a keen eye for detail and approaches problem-solving with a logical and methodical mindset.
Ambitious: Emily is highly motivated and sets high standards for herself. She strives for excellence in her academic pursuits and is determined to succeed in her chosen field. She actively seeks out opportunities for growth and is not afraid to take on challenging projects.
Curious: Emily possesses a strong intellectual curiosity and an insatiable desire to understand how the economy functions. She eagerly explores various economic theories and is always on the lookout for new research and emerging trends in the field. She constantly seeks to expand her knowledge beyond the classroom.
Collaborative: Emily recognizes the value of teamwork and believes in the power of collaboration. She enjoys engaging in discussions with her peers, sharing ideas, and working together to solve problems. Emily actively contributes to group projects and encourages open communication and brainstorming.
Detail-oriented: Emily pays close attention to details, ensuring accuracy and precision in her work. She thoroughly reviews her assignments and meticulously checks her calculations to avoid errors. She believes that small details can make a significant impact on the overall outcome.
Communication Style: Emily’s communication style is articulate and well-reasoned. She is confident in expressing her thoughts and ideas, both verbally and in writing. She is skilled at breaking down complex economic concepts into more understandable terms, making her an effective communicator in both academic and social settings. Emily actively listens to others and respects diverse viewpoints, fostering constructive and open discussions.
GPA: Emily maintains a consistently high GPA, typically ranging between 3.8 and 4.0. Her dedication to her studies and her disciplined approach to learning contribute to her academic success. She consistently demonstrates a strong work ethic and is known for her outstanding performance in economics-related courses. Emily takes pride in her GPA and strives to maintain her excellent academic standing.
[1] Camerer (2019) discussed AI’s impact on behavioral economics, stating it identifies behavioral variables influencing human behavior. Also, many experiments involve humans playing games against computers.
[2] Pre-trained transformer models for large corpora demonstrate their ability to solve various natural language processing tasks (Zhao et al., 2023).
[3] Kocoń et al. (2023) report that the bias in ChatGPT is most likely caused by the rules OpenAI imposed on human trainers.
[4] For example, consumer product reviews are only sometimes based on consumers’ true preferences. Brand et al. (2023) point out that this is an issue in using generative AI for consumer research.
[5] We can interpret this as different items being offered in each round of the auction. We would obtain more robust results if there were consistent trends in bids for different valuations.
[6] The optimal strategy in FPA refers to a symmetric Bayesian equilibrium strategy.
[7] This result suggests that ChatGPT may not distinguish between FPA and SPA auction rules.
[8] Bidders with more experience in real-world auctions tend to underbid (Garratt et al., 2012).
[9] Some research shows that human subjects learn little about optimal bidding strategies even as rounds proceed (Güth et al., 2003; Cooper & Fang, 2008).
[10] ChatGPT mentioned almost nothing about “why it chose that bid” in the SPA.
[11] See Appendix for the description of Emily.
References
Aher, G., R. I. Arriaga, & A. T. Kalai. (2023). Using Large Language Models to Simulate Multiple Humans and Replicate Human Subject Studies. arXiv preprint arXiv:2208.10264
Butler, S. (2023). How to Create ChatGPT Personas for Every Occasion. How-To Geek, Mar 28, 2023, 8:00 am EDT: https://www.howtogeek.com/881659/how-to-create-chatgpt-personas-for-every-occasion/
Brand, J., A. Israeli, & D. Ngwe. (2023). Using GPT for Market Research. Harvard Business School Marketing Unit Working Paper No. 23-062, Available at SSRN: https://ssrn.com/abstract=4395751
Camerer, C. F. (2003). Artificial Intelligence and Behavioral Economics. The Economics of Artificial Intelligence: An Agenda, ed. by A. Agrawal, J. Gans, and A. Goldfarb, The University of Chicago Press, Chicago and London, 587–608.
Cooper, D. J. & H. Fang (2008). Understanding Overbidding in Second Price Auctions: An Experimental Study. The Economic Journal 118(532), 1572-1595.
Cooper, A. & R. Reimann (2003). About Face 2.0 The Essentials of Interaction Design, Wiley.
Cox, J., B. Roberson, & V. Smith. (1982). Theory and Behavior of Single Object Auctions. Research in Experimental Economics Vol. 2, ed. V. Smith, Greenwich, CT: JAI Press, 1-43.
Cox, J., V. Smith, & J. Walker. (1988). Theory and Individual Behavior of First-Price Auctions. Journal of Risk and Uncertainty 1(1), 61-69.
Engelbrecht-Wiggans, R., & E. Katok. (2008) Regret and Feedback Information in First-Price Sealed-Bid Auctions. Management Science 54(4), 808-819.
Garratt, R. J., M. Walker, & J. Wooders. (2012). Behavior in Second-Price Auctions by Highly Experienced eBay Buyers and Sellers. Experimental Economics 15, 44-57.
Güth, W., R. Ivanova-Stenzel, M. Königstein, & M. Strobel. (2003). Learning to Bid—An Experimental Study of Bid Function Adjustments in Auctions and Fair Division Games. The Economic Journal 113(487), 477-494.
Horton, J. J. (2023). Large Language Models as Simulated Economic Agents: What Can We Learn from Homo Silicus? arXiv preprint arXiv:2301.07543
Kagel, J., & D. Levin. (1993). Independent Private Value Auctions: Bidder Behaviour in First-, Second- and Third-Price Auctions with Varying Numbers of Bidders. The Economic Journal 103, 868-879.
Kocoń, J., I. Cichecki, O. Kaszyca, M. Kochanek, D. Szydło, J. Baran, J. Bielaniewicz, M. Gruza, A. Janz, K. Kanclerz, A. Kocoń, B. Koptyra, W. Mieleszczenko-Kowszewicz, P. Miłkowski, M. Oleksy, M. Piasecki, Ł. Radliński, K. Wojtasik, S. Woźniak, & P. Kazienko, (2023). ChatGPT: Jack of All Trades, Master of None. Information Fusion 99, 101861. Zhao, W. -X., K. Zhou, J. Li, T. Tang, X. Wang, Y. Hou, Y. Min, B. Zhang, J. Zhang, Z. Dong, Y. Du, C. Yang, Y. Chen, Z. Chen, J. Jiang, R. Ren, Y. Li, X. Tang, Z. Liu, P. Liu, J-Y. Nie, & J. -R. Wen. (2023). A Survey of Large Language Models. arXiv preprint arXiv: 2303.18223
Relevant Articles
-
Japanese elementary teachers’ problem-based learning through online professional development on teaching Japanese language learners in physical education
by Takahiro Sato - 2024,S1
VIEW -
The Effects of Positive and Negative Ions on esports Performance and Arousal Levels Part 2 -Testing Higher Ion Density-
by Goichi Hagiwara - 2024,S3
VIEW -
Practical research on the use of digital pens in high school rugby club activities
by Katsuro Kitamura - 2024,S4
VIEW -
Transferability of Multiple Object Tracking Skill Training to Professional Baseball Players’ Hitting Performance
by Ryousuke Furukado - 2024,S5
VIEW